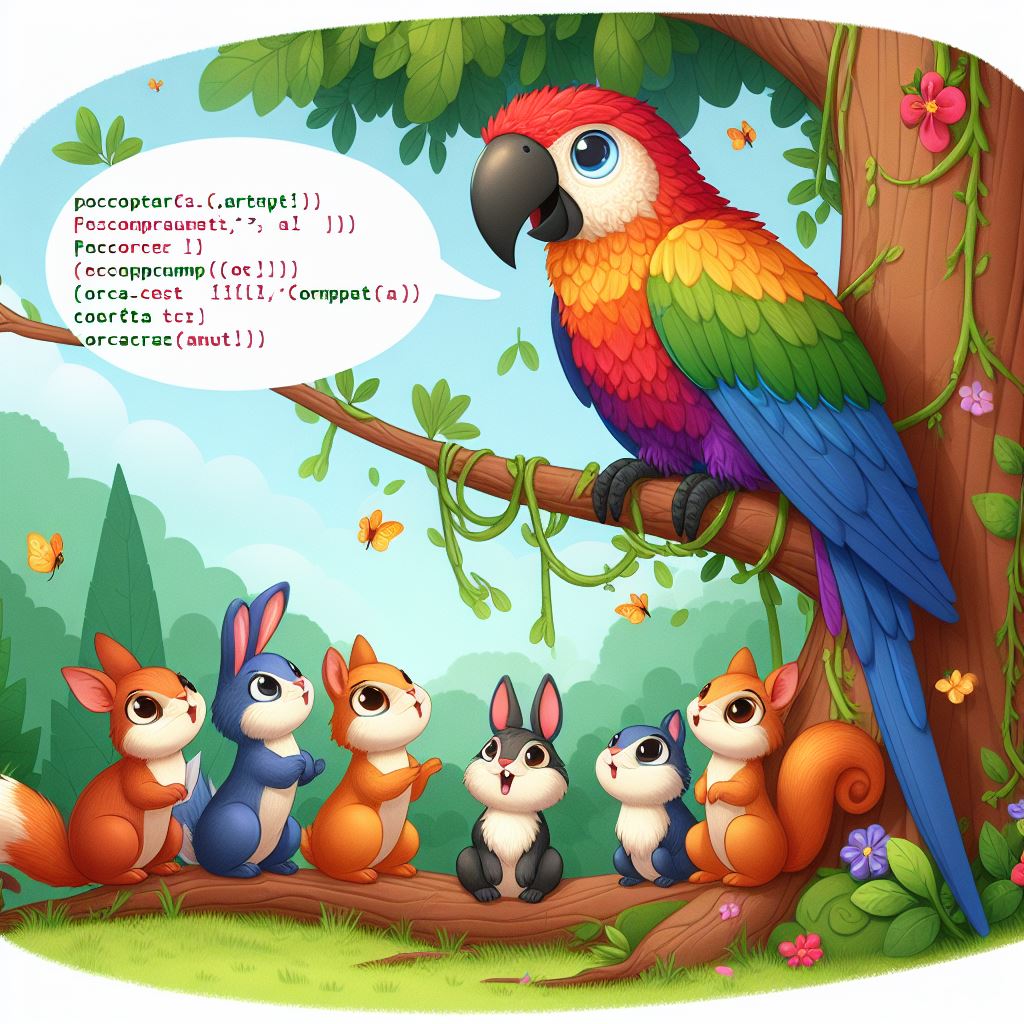
Building on some of my previous work on machine learning using and testing QDA Miner, NVivo, ATLAS.ti and Leximancer for analysing National Student Survey data. I’ve also developed some of this work as teaching datasets for ATLAS.ti, NVivo and MAXQDA through SAGE.
SRA Seminars
I was invited in December 2023 to contribute to a fascinating symposium organised by Dr Christina Silver and the Social Research Association – see my segment here on YouTube.
The two parts of the symposium – Part 1 (primarily developers and also an excellent discussion on the history and development of software for QDA with Susan Friese and Silvana di Gregorio) and part 2 with user perspectives which I was part of – talking about my work with machine learning and text mining in QDA software. (I return to this below based on a response to my segment.)
First what’s next:
SRA Conference Keynote
So I’m super-excited to be presenting a keynote with Christina Silver at the SRA Annual Conference 2024 building on that symposium. We’ve spent a LOT of time developing our presentation and cutting it down to size (so we’re planning a podcast in due course to re-surface all the stuff we had to cut out).
It’s been a fascinating and, at times, really challenging journey. We were spurred by the hype and some fasicnating and important critical insights into how AI is being promoted, positioned and boosted by the QDA software companies in this recent paper by Trena Paulus and Vittorio Marone:
Paulus, T. M., & Marone, V. (2024). “In Minutes Instead of Weeks”: Discursive Constructions of Generative AI and Qualitative Data Analysis. Qualitative Inquiry, 0(0). https://doi.org/10.1177/10778004241250065
Also Christina’s just-published podcast with Janet Salmons.
I’ve also been loving, doing a LOT of listening to, and citing, Emily M Bender’s contributions to the fantastic Mystery AI Hype Theatre 3000 podcast. All of it ithat is good to listen to, however for QDA I’d particularly recommend Episode 31 “Science is human” and especially Episode 28 “LLMs are not human subjects”.
Emily Bender was the author of the incredibly important stochastic parrots paper. A paper that is incredibly significant not just for its content, but what Google did to the co-authors Timnit Gebru and Margaret Mitchell in response to its publication. Which tells you a LOT about who’s voices are valued by the key developers and boosters of the current iteration of these technologies.
On criticality vs negativity (and the journey of the keynote presentation)
My fear is that in the SRA keynote I’m going to come across as negative about AI rather than critical of this first round and the hype, false promises, problematic discourses and ethical + environmental concerns. Some of what’s possible could be great, but to be great it needs to be quite different (and much less hyped).
We made this slide (from the image on this wikipedia page) but had to cut it due to time:

I definitely started being the figure bottom left – wowed in particular by ATLAS.ti’s implementation and the promise of their developments with “intetntional coding”. I saw the negative reception from some more traditional qualitative (academic) reseachers as being quasi-luddite. However, their concerns about publication restrictions are justified. And those are based on using unspecified LLM-powered authoring, but the applicaton to coding is the same tech doing a different task and again, you can’t recover nor clarify how the labels were created. So unlike the open-source and clearly documented text-mining algorithms that underpin other auto-coding in say QDA Miner/WordStat or the sentiment analysis and concept mapping in ATLAS.ti it’s a black box – or this:
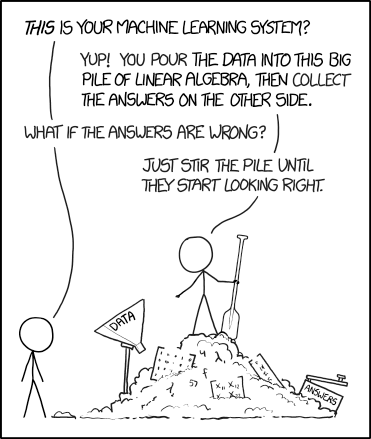
Which led to a lot more digging as well as experimenting with tools and just really thinking about issues – that was the climb up hype (aka: bullshit) mountain and I’m currently rapidly doing this down the steep slope expecting this. However, I am trying to look ahead to where that plateau of productivity might lie.
If I had one real hope it’s that LLMs could give me a really nice natural language interface to operate QDA MIner/Wordstat so what it was doing was recoverable and documented but I would be able to get an AI to translate my lay description of a statistical test into running the code/script for that test. Which brings me to…
On the response to my symposium segment in the Text Analytics Blog (and my reply)
There’s been a brilliant response with evidence and testing contesting some of my assertions by Normand Pelladieu of Provalis Research (who make QDA Miner).
I found the response fascinating. I also fully accept all of Normand’s critique and that I had under-estimated the pontential benefits and speed of local installations as well as the way they more easily comply with institutional data management policies. However, I do have a few thoughts.
Firstly, the test machine used is the sort of spec I dream of! I have a managed MS Surface with a 2.5Ghz i5 chip and 16Gb of RAM and only able to use locally synchronised OneDrive storage, so would LOVE to play with an Intel Core i9-10900 CPU, 64GB of RAM, and 4TB of disk space – but that’s never going to happen for me or any colleagues I know at a UK Uni’s!
Normand has subsequently clarified that this was basically giving the other softwsre a major leg-up as QDA Miner/word stat are only 32-bit – yet still wiped the floor with these other packages.
Secondly, I guess it’s not having that kind of computing power at my fingertips that makes me amazed at the benchmarks. I’m honestly stunned that NVivo didn’t just crash! (I can’t get it to work with datasets any larger than the NSS data – and my work on that was done with the last moderately stable release – v12. I consider R1 essentilaly unusable and v14 similarly problematic. I have yet to make it through a teaching session without NVivo crashing at least once as it leaks memory liek a seive and apparently relies on the system printer to render any results on screen! Top tip: if using NVivo restart your computer before you begin, and at least once or twice in the day – just closing it doesn’t release memory and it will leak until it crashes having used all your system resources. Plan for crashes, save often, backup often and try typing or coding with fingers crossed.
I’d love to see the OpenAI integrations benchmarked in the same way – my initial experiencs are that they are very, VERY (essentilaly unusably at scale) slow.
That QDA Miner ripped through the data doesn’t suprise me, and when I eventually get a copy installed on my managed laptop (it cant run on this M1 Mac) I look forward to trying to learn it again. However, I still think that the points about familiarity of interface and usability of software and training overheads have a huge significance in the selection and adoption of tools. This is often just a bias from the sunk-cost fallacy, but still a key consideration. While it would be possible to become the “Wordstat guy” and do really quick, more reliable, more clearly documnted and and by any metrics that count “better” work on tasks like the NSS comment analysis, it wouldn’t be possible to collaboarate with others in any institution I’ve had links with. By comparison NVivo did seamlessly build on existing skills and knowledge as well as allowing sharing. Sharing included not just the outputs/visualsiations/”results” as images in presentations and reports, but also supported sharing the underlying data and step-by-step guidance for anyone who wanted to look deeper (e.g. a head of department who didn;t want t take anyone else’s interpretation over their own).
I’m excited (but honestly: daunted and time-pressed) to try and re-acquaint myself with QDA Miner and try to wrap my head around WordStat, but I’m NOT a statisticin and, unlike Normand, my brain is not planet-sized.